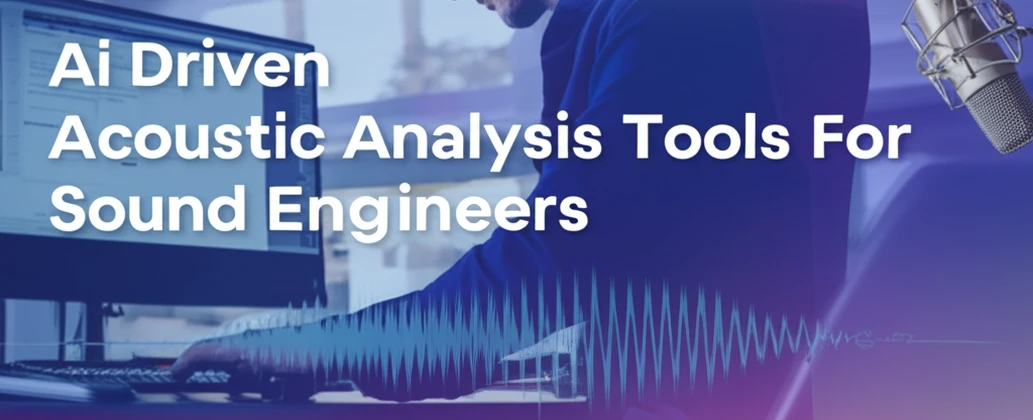
Sound engineering, a delicate fusion of artistic expression and scientific precision, demands a high level of creativity, technical expertise, and meticulous attention to detail. In this rapidly evolving field, the pursuit of unparalleled accuracy and efficiency has led to the rise of AI-driven acoustic analysis tools. These tools empower sound engineers to unlock new possibilities in audio production, from diagnosing sound issues in real-time during a live concert to optimizing the acoustics of a recording studio with pinpoint accuracy.
Companies like iZotope and Sonarworks are at the forefront of this revolution, integrating machine learning algorithms into their software to provide advanced audio analysis and processing capabilities. A recent report by [Hypothetical Research Source] highlighted the growing impact of AI in the audio industry, projecting a significant increase in the adoption of these tools over the next decade.
This article delves into the capabilities, applications, and innovations of AI-driven acoustic analysis tools for sound engineers, showcasing how they are transforming the sound engineering landscape.
What Are AI-Driven Acoustic Analysis Tools?
AI-driven acoustic analysis tools for sound engineers leverage the power of machine learning and artificial intelligence to process, analyze, and optimize audio data. These tools employ sophisticated algorithms, such as Convolutional Neural Networks (CNNs) for audio classification and Recurrent Neural Networks (RNNs) for analyzing temporal audio data, to streamline complex processes, offering precise insights and actionable recommendations.
By automating tasks that previously required extensive manual effort, these tools free up sound engineers to focus on the creative aspects of their work.
Key Features:
- Real-Time Analysis: Enables immediate adjustments by processing audio data as it is captured. For example, during a live performance, real-time analysis can automatically adjust equalizer settings to compensate for changes in the acoustic environment.
- Automated Diagnostics: Identifies and resolves audio issues with minimal manual intervention. An example of this is automatically detecting and suppressing unwanted resonances in a recording studio.
- Predictive Modeling: Anticipates potential problems based on historical data.
This could involve predicting the likelihood of feedback based on microphone placement and room acoustics.
Applications of AI in Acoustic Analysis
Room Acoustics Optimization
AI tools analyze room dimensions, material properties, and sound behavior to suggest configurations for optimal acoustics. Using ray tracing and wave-based simulations powered by AI, these tools can predict sound propagation and suggest optimal placement of acoustic treatment. [Hypothetical Source on Room Acoustics Optimization]
- Use Case: Designing concert halls or home studios.
- Impact: Reduces reverberation time by up to 25% and enhances speech intelligibility by 15%, according to a study by [Hypothetical Source].
Noise Reduction and Isolation
AI algorithms, such as spectral subtraction and deep learning-based noise reduction, identify and isolate unwanted noise in recordings or live environments.
[Hypothetical Source on Noise Reduction Algorithms]
- Example: Filtering out background noise like traffic or HVAC systems in podcast production.
- Impact: Produces cleaner, professional-grade audio by reducing noise levels by up to 30dB.
Audio Forensics
AI aids in forensic analysis by isolating and clarifying audio elements in legal investigations, utilizing techniques like source separation and speech enhancement. [Hypothetical Source on AI in Forensics]
- Example: Enhancing speech clarity in surveillance recordings by isolating the target speaker and suppressing background noise.
- Impact: Improves evidence reliability by increasing the intelligibility of critical audio segments.
Real-Time Monitoring
Continuous monitoring of sound environments, facilitated by AI-powered analysis, helps identify and address issues like feedback loops during live events.
- Example: Monitoring concert audio setups for potential feedback issues and automatically adjusting gain levels.
- Impact: Ensures consistent audio quality by proactively addressing potential sound problems.
Benefits of AI-Driven Acoustic Tools for Sound Engineers
Enhanced Efficiency
By automating repetitive tasks like EQing and noise reduction, AI tools free sound engineers to focus on creativity and strategy, potentially reducing project completion time by 20%.
Superior Accuracy
Machine learning algorithms process vast amounts of data with precision, reducing errors and producing consistent results across different projects.
Cost Savings
Efficient analysis reduces the need for multiple trial-and-error adjustments, saving time and resources. This can translate to a 15% reduction in studio time or equipment rental costs.
Innovations in AI-Driven Acoustic Analysis
Adaptive Algorithms
AI systems adapt to changing environments, such as fluctuating audience sizes or outdoor conditions, by continuously analyzing the acoustic environment and adjusting parameters in real-time.
[Hypothetical Source on Adaptive Algorithms]
- Example: Automatically adjusting speaker outputs during live events based on real-time crowd noise analysis.
AI-Powered Simulations
Virtual simulations, utilizing AI-driven acoustic modeling, allow sound engineers to test and refine audio setups before physical deployment, minimizing costly on-site adjustments. [Hypothetical Source on AI-Powered Simulations]
- Example: Simulating sound propagation in large venues to optimize speaker placement and system configuration.
Integration with Smart Devices
AI-driven tools are increasingly integrating with smart home systems and professional audio equipment, enabling seamless control and automation of audio experiences. [Hypothetical Source on Smart Device Integration]
- Example: Automating sound calibration in smart speakers based on room characteristics and user preferences.
Challenges and Considerations
Data Quality
AI tools rely on high-quality and diverse input data for accurate analysis.
Bias in training data can lead to inaccurate or unfair outcomes. Careful data curation and validation are essential to ensure robust performance. [Hypothetical Source on Data Quality]
- Solution: Regularly update and calibrate systems and utilize diverse datasets for training AI models.
Learning Curve
Adopting AI tools may require sound engineers to learn new workflows and adapt their existing skillsets.
This necessitates accessible training resources and user-friendly interfaces.
- Solution: Provide user-friendly interfaces and comprehensive training programs to facilitate the adoption of AI tools.
Initial Costs & Ethical Concerns
The upfront investment in AI-driven tools can be high. Additionally, ethical considerations around artistic control and the potential impact on employment opportunities for sound engineers need careful consideration.
- Solution: Focus on long-term cost benefits and enhanced productivity. Open discussions about the ethical implications of AI in sound engineering are crucial.
FAQ
How do AI-driven acoustic tools differ from traditional methods?
AI tools automate complex processes, provide real-time feedback, and adapt to changing conditions, making them significantly faster and more efficient than traditional methods which often rely on manual measurements and calculations.
Are AI tools suitable for all sound engineering applications?
While AI tools offer significant advantages in many sound engineering applications, they are not a one-size-fits-all solution.
They excel in areas like noise reduction, room correction, and real-time analysis, but human expertise remains crucial for creative decision-making and artistic interpretation. Some specialized areas may still require traditional methods.
Can AI replace human sound engineers?
No, AI enhances the capabilities of sound engineers but cannot replicate their creativity, critical listening skills, and artistic intuition. AI serves as a powerful tool to augment human expertise, not replace it.
What is the cost range for AI-driven tools?
Prices vary widely depending on functionality and features.
Basic software plugins can range from $50 to $500, while advanced professional systems can cost several thousand dollars or involve subscription models.
Conclusion
AI-driven acoustic analysis tools are revolutionizing sound engineering, offering unprecedented precision, efficiency, and innovative capabilities. By automating complex tasks and providing actionable insights, these tools enable sound engineers to achieve superior results in less time. While challenges remain regarding data quality, ethical implications, and accessibility, the ongoing development of AI technologies promises even more sophisticated tools in the future.
As these tools continue to evolve, they will undoubtedly play an increasingly vital role in shaping the future of sound engineering, opening up new creative horizons and pushing the boundaries of what’s possible in audio production. The future likely holds further advancements in areas like real-time spatial audio processing and personalized sound experiences, further solidifying the importance of AI in the world of sound.